Advancing Data Literacy
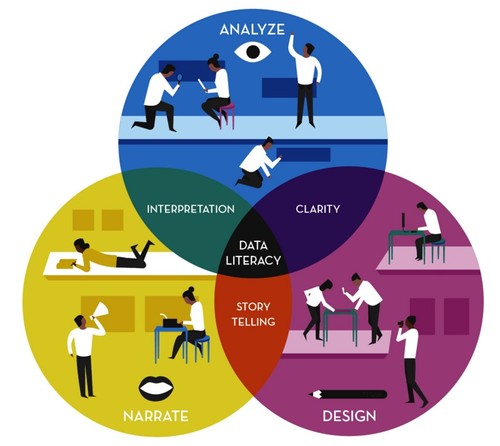
To deepen the benefits of Big Data, we must put the social sciences and the humanities on equal footing with math and computer science.
Outside the typical Big Data tropes—gathering, storing, measuring, parsing, analyzing—I want to argue that contextualizing, storytelling, and visualizing are equally important tools to help us understand, describe, and put to Big Data to work. I define Data Literacy as the interplay between the typical tropes and the added layer of the social sciences and humanities.
To be clear: Data Literacy isn’t as simple as the ability to store, command, and read numbers. And it isn’t something that is possible to achieve through just one person.
Rather, on the production side, it’s a process that involves different competencies at each step; on the consumption side, it’s an acumen in judging the credibility of a final product. On both sides it’s an understanding of the fundamental problems that can crop up along the way, from strategy to data collection to filtration to analysis to presentation:
The things we want to measure, but don’t know what data to collect.
The data we want to collect, but don’t know how to capture.
The data we’ve captured, but don’t know how to interpret.
The data that we misinterpret, because there’s too much noise and not enough signal.
The data that we misattribute, because we mistake correlation and causality.
The data that we misuse, because we want them to support an agenda based on falsehoods.
Without Data Literacy, we end up in one of the following scenarios with regard to Data:
we don’t collect it;
we ignore it;
we look at it, but don’t apply it;
we apply it incorrectly;
we extract the wrong meaning from it;
or twist it to support our (wrong) ideas.
Data Literacy can help us solve those problems, but it’s only one part of the puzzle.
Anyone can throw a few numbers together to make a quick statistic, or compile tons of them into massive spreadsheets, but without any real meaning to be extracted we’re left with numerical gibberish, or “data salad,” if you will. This is where contextualization, narration, and design / visualization come into play; described for the purpose of how we can enable Data Literacy below.
Contextualization is a process of putting findings into perspective. It is a tool that social scientists — sociologists, anthropologists, economists, political scientists, psychologists, geologists, historians, archaeologists — put to work in order to better understand what they want to know, how to go about answering it, and what their findings need to consider to be as accurate as possible. It's also something we use a lot here at Made by Many.
Contextualization can also been seen as a powerful outcome created through the humanities and their use of philosophy, literature, religion, art, music, history, culture, and language to understand and record our world.
Even with those powerful endorsements, contextualization is also a tool that isn’t used nearly enough when data is analyzed and mined for insights — let alone considered when decisions are made around which data to collect (or not) in the first place. Keying in on the nuances under master-status-level things like gender, identity, education, race, religion, family history, personal experiences, and geography is critical when you are looking at controlling for or minimizing biases.
Employing methodologies and frameworks from the social sciences and humanities can get at key questions like:
- Who created the data, for what reason, under what conditions, for which purpose? What are the barriers, entry points, and backgrounds that impact their ‘data exhaust’?
- Who is gathering, analyzing, interpreting, explaining, and visualizing the data — what are their goals, seen and unseen biases, and personal backgrounds they bring to bear on these exercises?
- Who the ultimate audience or audiences? What framing do you have to employ to best communicate the findings — and what happens if they don’t understand or agree?
- What impact do things like the current zeitgeist, their geopolitical position in the world, or previously held beliefs play in the audience’s willingness to engage? Ability to understand?
Made by Many is one of the first companies that I’ve worked at that doesn't pride itself in having all the answers. Rather, its interdisciplinary approach means we certainly know how to ask the right questions. Here’s to advancing Data Literacy one new discipline, new voice, new method, and new question at a time.
This post is part of my ongoing thinking on the topic, which I’ve written about for Medium and other outlets. Please challenge me or add your thoughts!
Continue reading
Unbillable Hours: The Hodgepodge Edition
Unbillable Hours is our weekly round-up of what we’re doing when we’re not working on client projects, plus our most-talked-about Slack links.
Unbillable Hours: A peek inside the design channel
Unbillable Hours is our weekly round-up of what we're doing when we're not working on client projects, plus our most-talked-about Slack links.
Welcome to...
Want to see the new Hackaball?
Now on Kickstarter: https://www.kickstarter.com/projects/hackaball/hackaball-a-programmable-ball-for-active-and-creatLast year we made a working prototype...